Content Warning
Mitigating Bias in Machine Learning
Edited By @drcaberry
Brandeis Hill Marshall
„We dedicate this work to the diverse voices in #AI who work tirelessly to call out bias and work to mitigate it and advocate for #EthicalAI every day.
Some of the trailblazers doing the work are
@ruha9
@timnitGebru
@cfiesler
Joy Buolamwini
@ruchowdh
@safiyanoble
We also dedicate this work to the future engineers, scientists, and sociologists who will use it to inspire them to join the charge.“
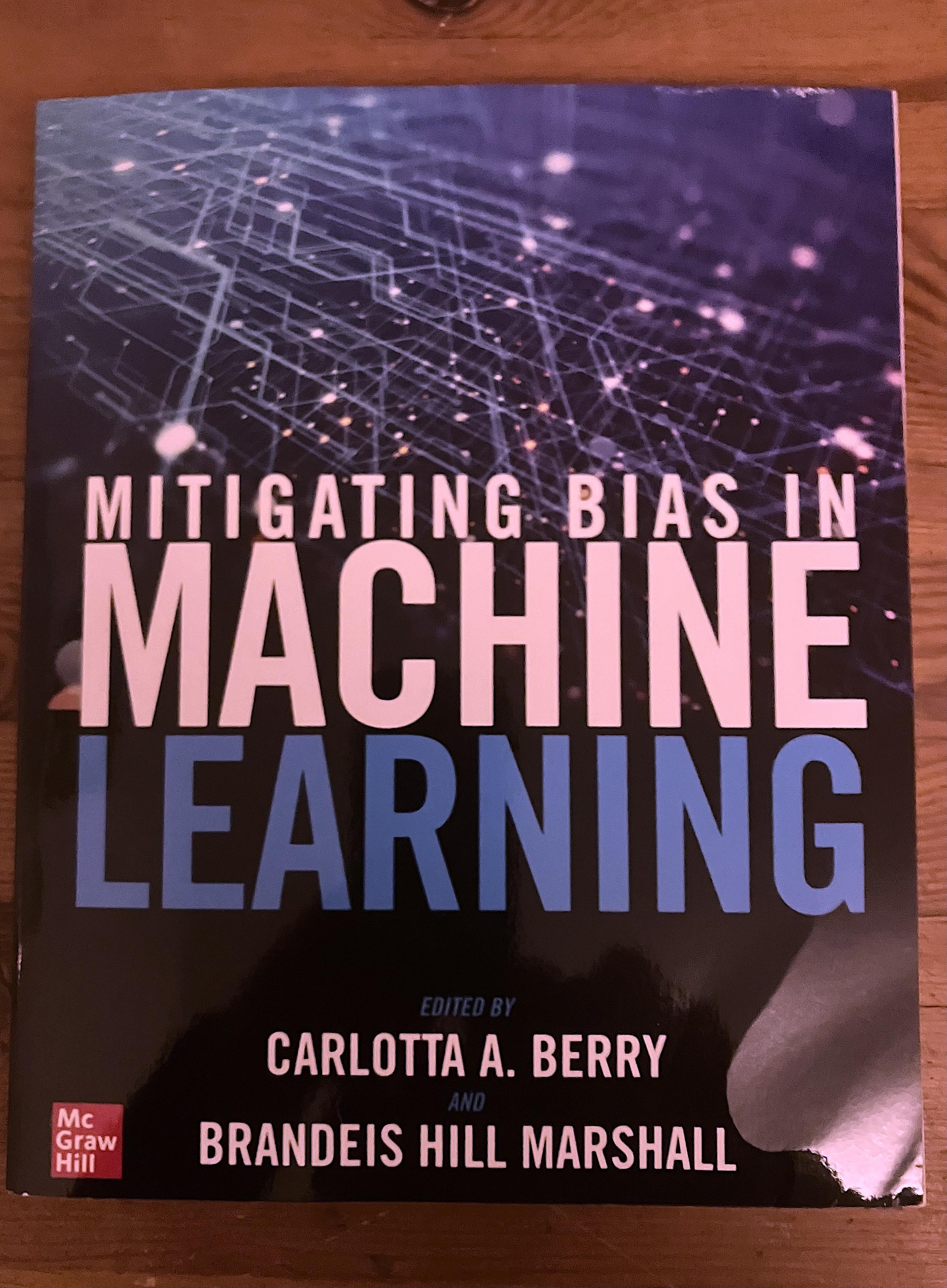
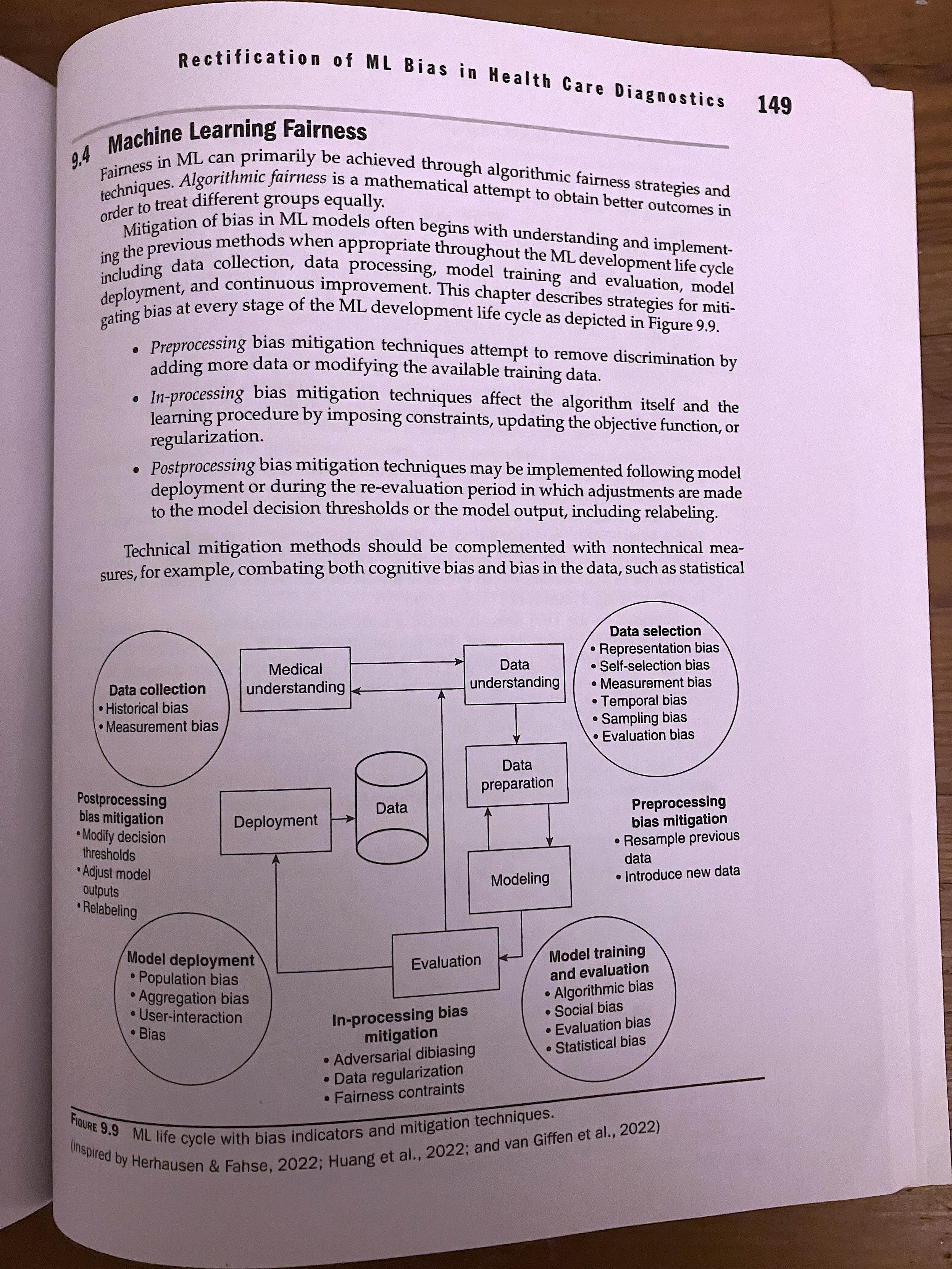